Getting More Insight from Cube Analysis
17 Sep 2015 | by Chris Roe
Breaking down your data into easily analysed dimensions or interesting segments can provide you and your team with invaluable insights into your data. This blog post explores how you can get the most out of your data in this way using the powerful analytical “Cube” tool within FastStats Discoverer.
What are Cubes?
Cubes are a powerful analytical tool for gaining insight from your data. Breaking down the data by multiple dimensions gives the analyst a way of looking for interesting segments. Using date dimensions allows us to see what is happening over time. Adding different measures based on numeric transactional values can give insight into valuable segments in the data.
Examples of insights provided...
There are some questions we could ask which require producing figures which are calculated from those returned by the analysis. For example:
- What is the difference in the average holiday spend compared to this quarter last year?
- How is the relative popularity of destinations to each other changing over time?
- What is the sum of our revenue for the year to date?
In the cube below, we are looking at the different destinations visited over a 2 year period banded into quarters. It shows the number of people in each cell and also the average cost of their bookings for that destination/quarter combination.
To answer question (1) above we could set the following definition to compare the Mean(Cost) to value from the year before:
Then the results on the cube would look like this:
From the portion of the cube that we can see above it is clear that for the four visible destinations our average holiday spend in each quarter of 2012 was better than in 2011 (with only one exception).
Questions (2) and (3) posed earlier in this blog can be answered in a similar way by defining a measure that is calculated from information that already exists within the cube.
How will this capability be included in the latest release of FastStats Discoverer
For the Q3 2015 release of Discoverer we have added functionality to expand the analytical capabilities of cubes with a number of new ‘Calculated Measures’.
The following new functions are available:
- Running – cumulative function across a particular dimension on a measure.
- Rolling – smoothing fluctuations in the data by calculating a function across the last few values.
- Period To Date – work out the value of a function across a date dimension from the start of a date period.
- Comparison – compare the current value to a previous value on a dimension. For a date dimension this can compare to a relative date period.
- Rank – compare the relative position of a category amongst other categories across a dimension and return as a number.
- Percentile – compare the relative position of a category amongst other categories across a dimension and return as a percentage showing the number of values lower than or the same as the current one.
Furthermore, these useful calculations can be chained together to create more advanced results - for instance we could work out the difference between the Sum of the Year to Date against the Sum of the Previous Year To Date.
These new functions will add a lot of power for the analyst to be able to investigate questions that were not previously possible within Discoverer.
Key Takeaways:
- Breaking down the data by multiple dimensions enables in-depth analysis of interesting segments.
- Data calculations can be chained together to create even more advanced results
- The new functions of Discoverer will enable you to obtain an ever deeper level of analysis from your data.
Gain even deeper insights into your data and customer base by extracting meaning and sentiment from customer reviews & social media: Words Worth: Extracting Meaning and Sentiment from Textual Data
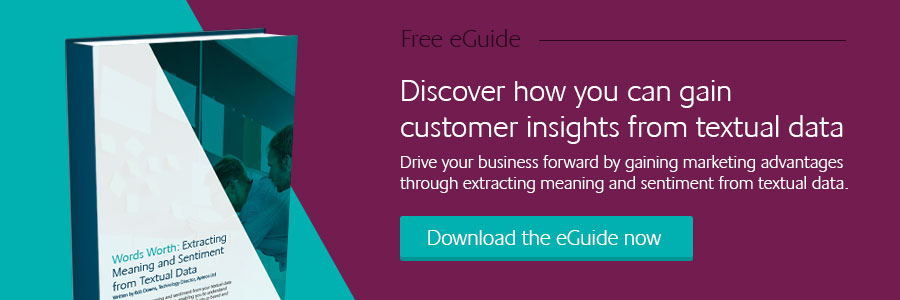